Explaining AI:
Designing to foster trust
Research and developing design principles to build explainable artificial intelligence that users trust.
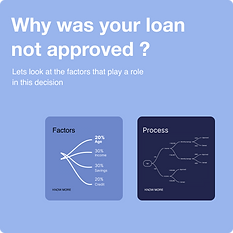
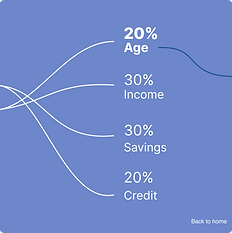

Time: 15 weeks (July - October, 2020)
Team: The project team comprised of Dr. Sumitava Mukherjee, PI of the Decision Lab, Deeptimayee Senapati, a cognitive sciences PhD student at IIT Delhi, and me.
My Role: Collating and synthesizing secondary research for the PI, building and deploying surveys, analyzing data and gathering insights, representing the team to present our findings at the Annual Conference of the Society of Judgment and Decision Making.
Methods: Literature review, survey research, statistical analysis
Tools: Google forms, SPSS (statistical package for the social sciences)
In Summer 2020, I worked as an intern at the Decision Lab - a cognitive sciences and HCI research lab at the Indian Insititute of Technology, Delhi. I worked on a research project that explored factors that influenced human perception of algorithmic judgment to provide directions for future development of explainable AI and fostering trust in artificial intelligence.
Scope
Overview
What
Our research focused on finding the factors that influence public trust in artificial intelligence and algorithms. We aimed to gain more insight into the how an individual’s perceived understanding of the system and the domain in which the algorithm works affects their trust towards the system.
Why
Artificial intelligence and algorithms are being rapidly integrated into our daily lives. However, research has found that people tend to be skeptical about algorithmic judgments. It is necessary to ensure that AI integration is met with public acceptance.
How
We conducted literature review to pin point the various factors that influence human judgment of artificial intelligence. Further, we conducted survey research to understand the influence of perceived understanding and domain specificity as factors affecting trust in AI.
The Problem
Despite the proven efficacy of artificial intelligence algorithms, people are still aversive towards algorithmic decisions.
Before beginning our primary research, we found it useful to go back to existing literature about algorithm aversion to identify research gaps and possible avenues for primary research.
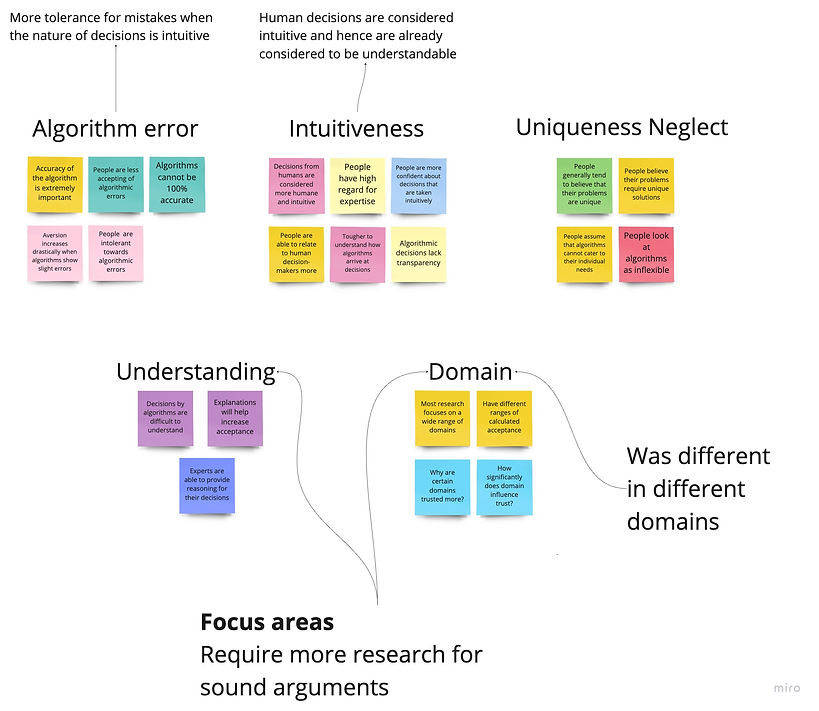
Through clustering, we found that while multiple factors affected human judgment of algorithms, studies show disputed results in the way they influence people’s trust.
A closer look into the details of the studies showed that each of them worked with different domains and had different interactions with the users. We decided to focus on these aspects.
Survey Research
From this project, we were aiming to understand the cognitive factors that affect acceptance of artificial intelligence in the Indian population. Hence, we planned to do a large scale survey research to validate our hypotheses.
Since our focus lay on the integration of AI algorithms in the public, our target population included anyone who would interact with the algorithms. Thus, the participants came from different educational, professional, and cultural backgrounds.
We used a convenience sampling method to recruit participants. The survey was posted in online forums, discussion boards, and university learning management systems where people were asked to fill it.
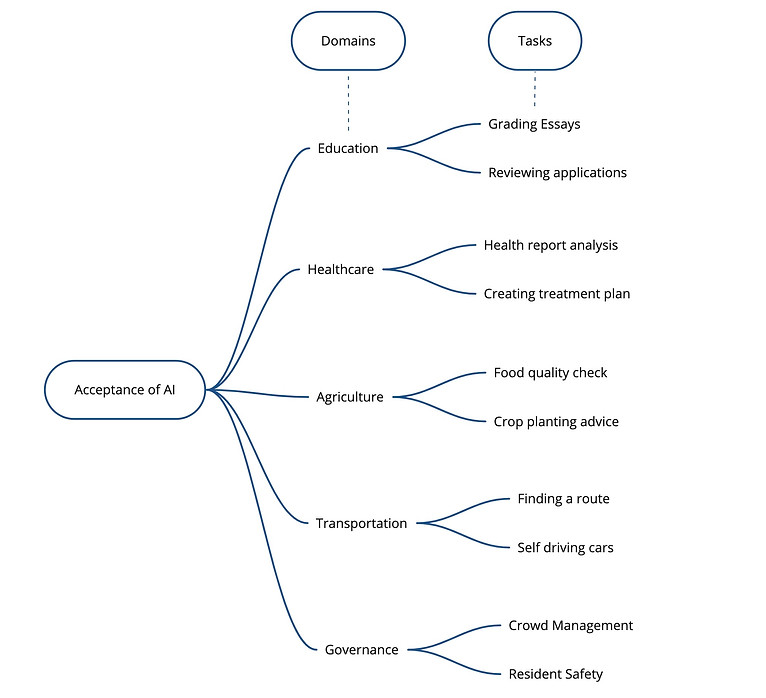
Domains and tasks used in the survey
The survey was designed on the basis of the Technology Acceptance Model and covered 5 domains - education, health care, agriculture, transportation, surveillance and governance. In each domain, individuals were given a hypothetical scenario where they have to respond to an AI making decisions for them. To avoid any form of external bias, we felt it was important to keep the scenarios hypothetical.
We coded the questions based on perceived understanding, acceptability, nature of task, and preference between human and AI decisions and quantified it on a 10 point Likert scale.
Through random and convenience sampling, we conducted the survey on 193 participants. The responses were analyzed through SPSS and regression analysis.
Key Insights
Our research showed that trust in AI decisions is driven primarily by these factors:
01
Trust in algorithm depends on the domain of the algorithm.
For the students, using the application outside of class meant practicing extra problems. The lack of problems on the web application deterred them from using it.
03
Familiarity with domain has an influence on trust
Due to remote learning, it is difficult to help students learn how to use the app during class. Given the time constraint, the professor is only able to go over the basics. Without accurate guidance students will not use the app outside class.
02
People's judgments about algorithms tend to vary according to the objectivity of the task
Most view the application as an isolated way of learning - they are unsure whether they are doing the problem correctly, but have no one to discuss it with.
04
An increase in perceived understanding increases the acceptability of the system
Since students are not using the app outside of class, they are not able to independently work out problems in an applied manner. This reduces the impact of the tool as the goal of using it is not achieved.
Design Principles
Based on these insights, I formulated some design principles for designing explainable AI that will help foster trust in the systems
1. Giving context of the domain
2. Showing the objectivity of the task
Along with the context of the specific tasks, giving the user context of the domain and the way decisions are made in it will help the users understand the nature of the tasks better and the steps involved in the process. This will help increase acceptability of algorithms taking those steps.
The tasks need to presented in an objective manner - breaking down the tasks into steps and presenting that will help users accept that an algorithm will be able to perform it.
3. Simplifying for better understanding
The descriptions provided need to be simple and not overcomplicated. Humanizing the explanations by explaining technicalities through examples will help to increase understanding and foster trust.
Designing Explainable AI
In order to understand how these design principles can be implemented, I took an example of a loan processing AI machine and its explanation. I redesigned the explainable AI according to the insights derived from the research.
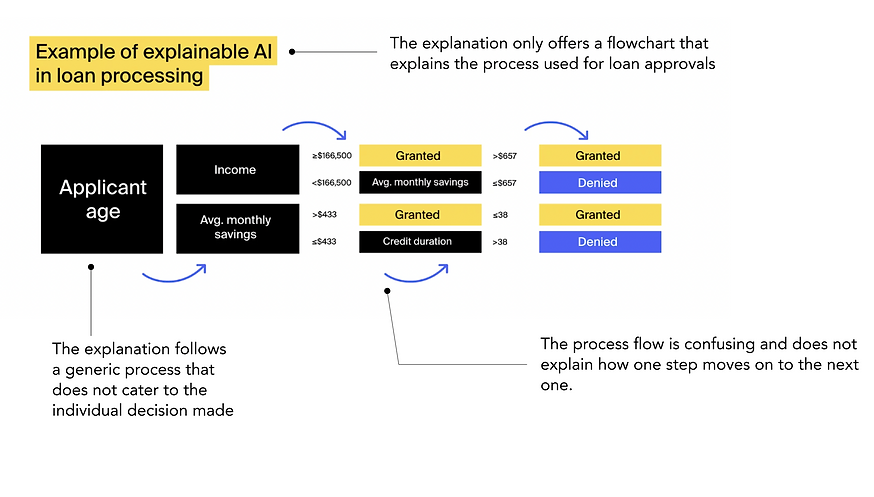
Example of the AI explanation used and its flaws
Based on the design principles, I created an interactive prototype of the explainable AI
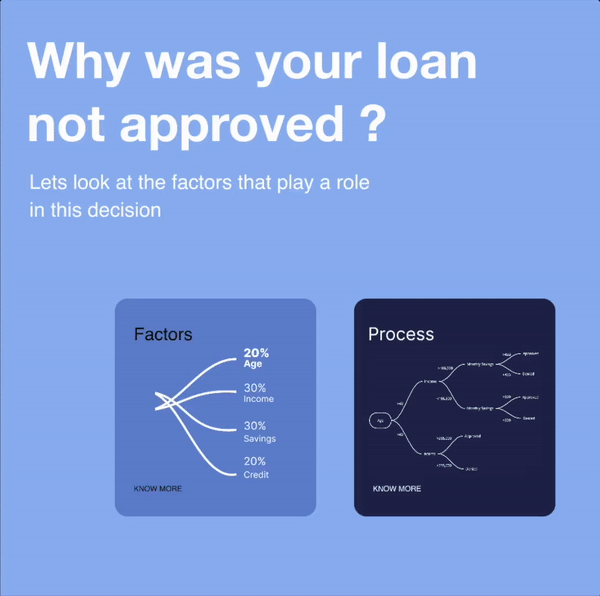
DESIGN DECISIONS
Instead of providing the complex algorithms which are incomprehensible for lay-persons, the explanation provides a simplified version of how each factor weighs into the decision.
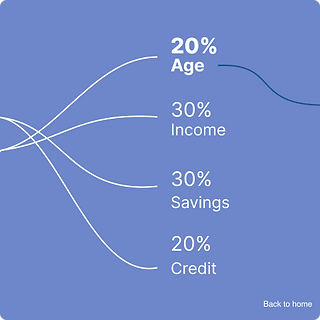

The explanation also provides a point of comparison to other demographically similar people that helps the user understand how to change the decision in the future and increase their chances.
A step-by-step process helps the user understand the decision made a series of smaller, fact-based decisions that can be quantified.
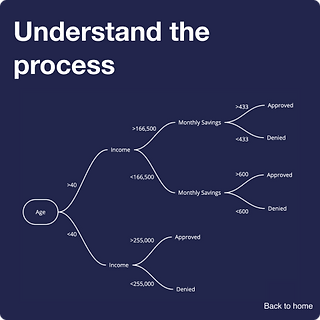
Reflections